Best Practices
Conversations: Mike Bushman on the Best Generative AI Use Cases for Events
23 Sep 2024 | Jessica F. Lillian | 4 minutes
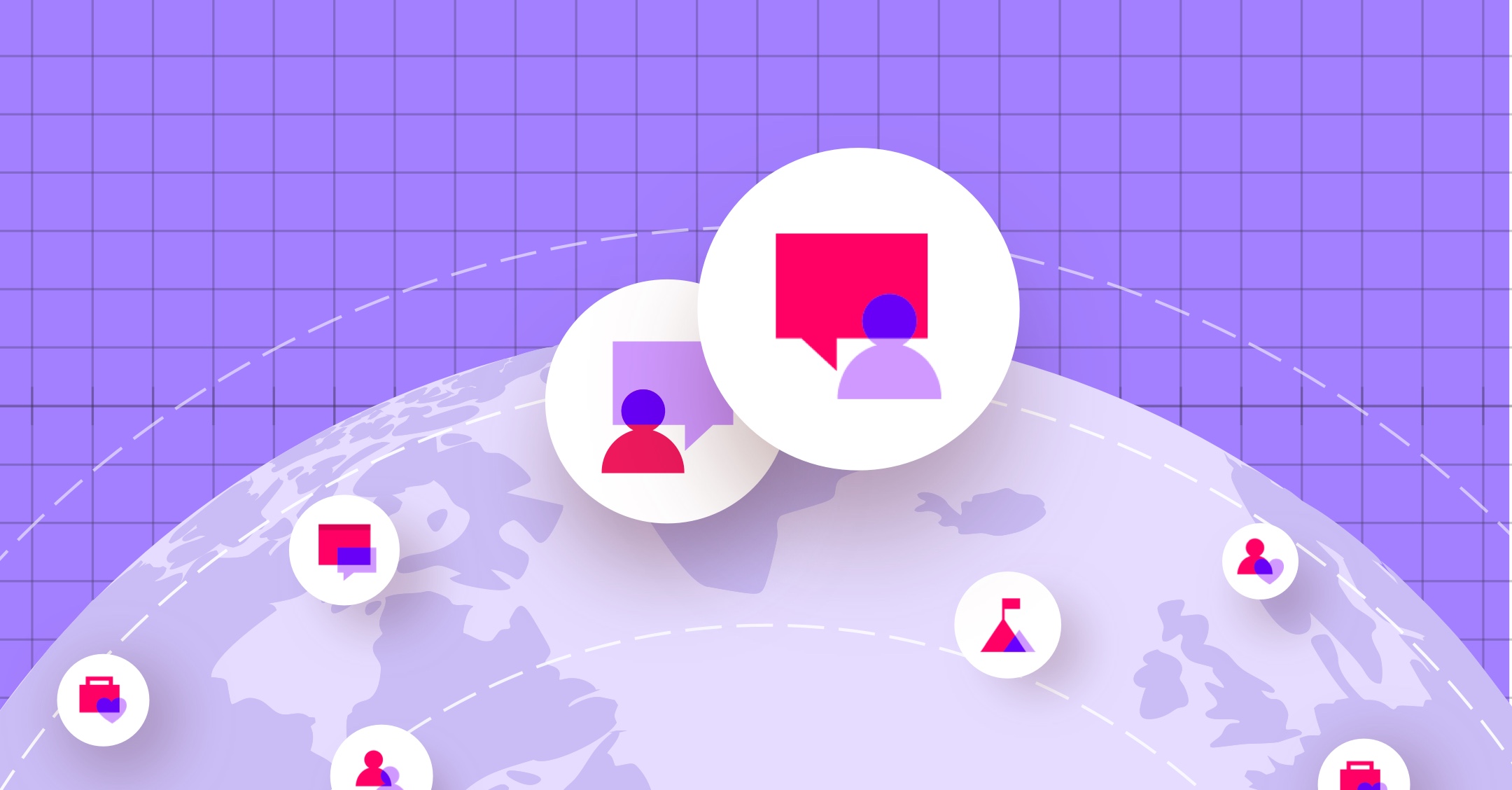
By popular request, RainFocus CTO Mike Bushman is back for another round of helping events and marketing teams make sense of the latest advancements in generative AI!
In our most recent conversation, we discussed critical issues around trust, transparency, and the entry of several major companies. Now, we’re taking a deeper look at the most compelling use cases so far. You’ll also learn how RainFocus approaches the process of incorporating potential new generative AI features in the platform.
For events, what are the main generative AI use cases you’re seeing among clients right now?
As these models’ natural language capabilities have improved, we see clients using generative AI to write emails and session content, and automatically take notes in meetings. They’re also useful for extracting meaningful data from unstructured data. For example, with surveys, people are using AI for sentiment analysis, categorization, word clouds, and summaries.
Helping attendees find the right content at events is always top of mind with our customers. Generative AI can help here to suggest session tagging, categorization, and cohorts of sessions. These can be used to augment and improve the filters already in use in the session catalog.
We’ve also run more traditional — not generative — AI through machine learning to provide personalized recommendations for at least four large events so far this year. This personalization is driven by attendees’ interests, activities, and cohorts.
In our recent event content survey, we found that the most popular generative AI use cases among event teams were relatively simple tasks with transcription and content writing. Do you anticipate a move to more sophisticated tasks as the tech improves and users gain additional trust?
One other interesting use case we’ve seen already is for session scheduling, which can involve some very sophisticated algorithms. You can suggest and schedule a list of sessions automatically for the attendee. This list can be a curated list or an AI-personalized list from the recommendation engine.
On the data exploration side, a lot of people are looking for timely answers to their common event management questions. They include things like “How many people have registered this week?” or “How many approved speakers have uploaded their bio?” Generative AI can easily find the reports to answer these questions and then suggest similar reports that may also be of interest.
True data exploration can also happen through the use of natural language to automatically generate reports. This can be done safely using our metadata layer that takes into account data access permissions and roles. In essence, people can ask questions or use our report builder and get meaningful data from it.
There’s definitely so much possibility still to be explored! How would you describe RainFocus’ overall evaluation and testing strategy for these technologies, especially within the product and developer teams?
Our approach to incorporating potential generative AI components has been to position the technology as an additional tool that our product teams can use in their existing work, rather than creating a separate team. This ensures everything is well integrated and secure from a data privacy perspective.
Within the platform infrastructure and architecture, we have several core AI functional services: content generation, personalization, sentiment analysis, summarization, clustering, and RAG chatbots. Developers have all core services at their fingertips. For possible additional use cases, there’s a development AI board they can talk to. We’re always looking to enable the product team.
Anything else you’ve seen from clients so far? What additional recommendations and guidance would you give for future generative AI use cases?
One thing there’s been a lot of interest in is acceptable use policies for AI within a company. There’s a need to determine what tools are being used and what companies are involved. For us, the fact that we’re on a major cloud provider helps people feel more comfortable.
In addition to security, understanding costs for generative AI early on is important. For example, training your own model is quite expensive. It isn’t a valuable use of resources in most cases. Zero-shot and single-shot learning is usually sufficient. There will be an inherent cost for every form of usage, but measuring that from the start and building in a way to get maximum value is important.